· Solveion Insights · AI Strategy · 3 min read
Beyond Keywords: Mastering Prompt Engineering for Strategic Advantage
Unlock the true potential of Large Language Models. Effective prompt engineering is not just about asking questions; it’s about architecting conversations to drive predictable, high-value outcomes.
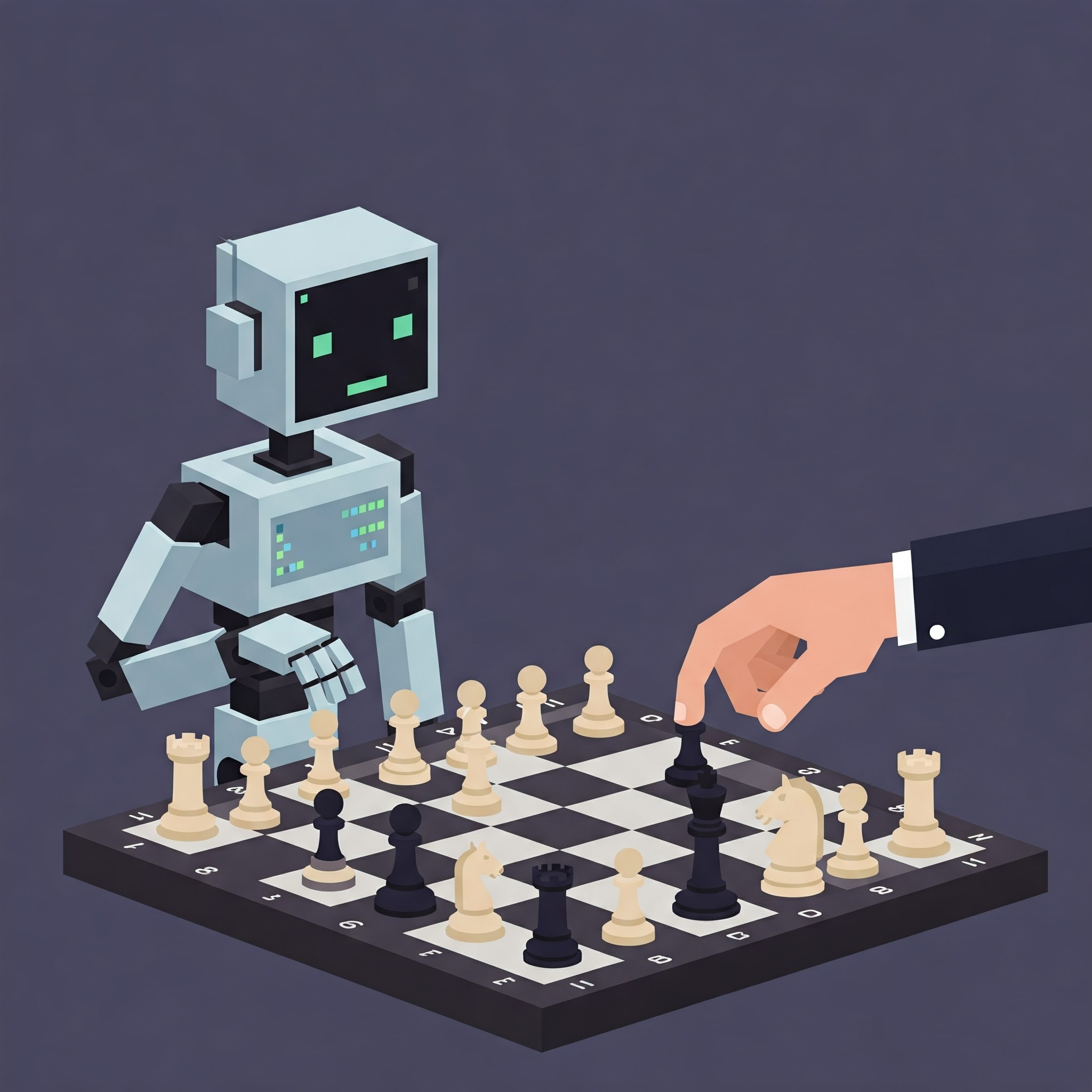
In the rapidly evolving landscape of Generative AI, the quality of interaction with Large Language Models (LLMs) directly dictates the value derived. While basic prompting yields basic results, mastering prompt engineering transforms these powerful tools from mere curiosities into strategic assets capable of enhancing productivity, creativity, and decision-making. This is not merely about finding the right keywords; it’s about understanding the model’s cognitive patterns and architecting conversations for optimal performance.
The Imperative for Precision: Why Basic Prompts Fail
LLMs operate on complex statistical patterns learned from vast datasets. They lack true understanding and rely heavily on the structure and context provided in the prompt. Ambiguous or poorly structured prompts lead to:
- Generic or Irrelevant Outputs: The model defaults to common patterns, failing to address specific needs.
- Inconsistent Results: Minor variations in phrasing yield drastically different outcomes.
- ”Hallucinations”: Confidently stated inaccuracies stemming from misinterpreted instructions or knowledge gaps.
- Inefficiency: Multiple iterations are required to achieve the desired result, wasting time and resources.
Simply put, treating an LLM like a search engine severely limits its potential. Strategic prompt engineering elevates the interaction from simple Q&A to a guided reasoning process.
Framework for Advanced Prompting: Key Principles
Moving beyond basic instruction requires adopting a structured approach. Consider these core principles, analogous to defining requirements in traditional consulting engagements:
1. Context is King
Provide sufficient background information. What domain are we operating in? What prior knowledge should the model assume? Explicitly state constraints, objectives, and the desired format of the output. Example: Instead of “Write about AI benefits,” try “Acting as a business strategist advising a retail CEO, outline three key benefits of implementing generative AI in customer service, focusing on ROI and customer satisfaction metrics. Format as a concise memo.”
2. Define the Persona and Role
Instruct the model to adopt a specific role (e.g., “You are an expert financial analyst,” “You are a creative copywriter specializing in tech startups”). This primes the model to access relevant stylistic and knowledge patterns.
3. Structure for Clarity
Use clear headings, bullet points, or numbered lists within your prompt to break down complex requests. Utilize delimiters (like ###
or '''
) to separate instructions, context, examples, and input data.
4. Employ Advanced Techniques
Explore methods like Chain-of-Thought (CoT) prompting (“Think step-by-step…”) to encourage logical reasoning, Few-Shot learning (providing 2-3 examples of desired input/output pairs), or ReAct (Reasoning + Action) frameworks for tasks requiring tool use or external information retrieval (often relevant in RAG systems).
5. Iterate and Refine
Prompt engineering is rarely perfect on the first attempt. Analyze the model’s output critically. Identify weaknesses or deviations from the instructions. Refine the prompt based on observations – clarify ambiguity, add constraints, or provide better examples. Treat it as a continuous improvement cycle.
Strategic Implications for Business
Investing in prompt engineering capabilities yields significant returns:
- Increased Productivity: Automate complex writing, analysis, and coding tasks more reliably.
- Enhanced Creativity: Use LLMs as sophisticated brainstorming partners and content generators.
- Improved Decision-Making: Leverage models for data synthesis, scenario modeling, and risk assessment with greater accuracy.
- Consistent Brand Voice: Define personas and instructions to ensure AI-generated content aligns with company style guides.
- Foundation for Custom Solutions: Well-engineered prompts are crucial for developing reliable custom AI applications and workflows, such as those utilizing RAG.
Conclusion: Architecting the AI Conversation
Effective prompt engineering moves beyond simple requests to architecting intelligent conversations. It requires clarity of thought, structured communication, and an iterative mindset. By mastering these principles, individuals and organizations can unlock unprecedented value from generative AI, transforming it from a novel technology into a core engine for strategic advantage.
Solveion provides expert training and consultancy to help your team master prompt engineering and integrate generative AI effectively. Contact us to learn more.