· Solveion Insights · AI Capabilities · 3 min read
Unlocking AI Reasoning: From Pattern Matching to Problem Solving
Beyond generating text or images, the next frontier for AI lies in its ability to reason. Understanding and cultivating this capability is crucial for developing truly intelligent systems.
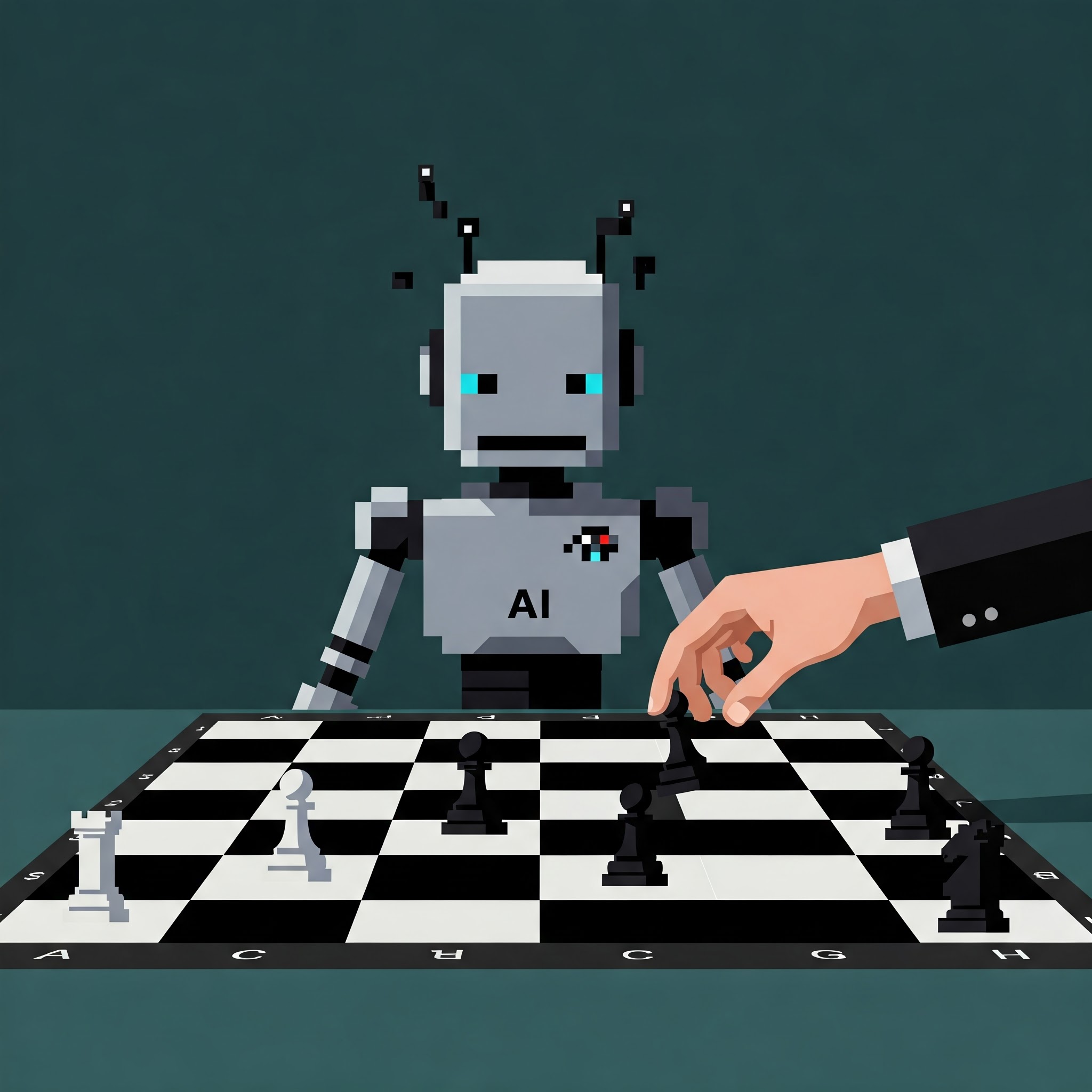
Current Large Language Models (LLMs) excel at pattern recognition and generation, tasks underpinned by sophisticated statistical analysis of vast datasets. However, achieving true artificial general intelligence (AGI), or even highly capable narrow AI, requires a leap beyond pattern matching towards genuine reasoning. This capability – the ability to logically process information, draw inferences, solve novel problems, and understand cause-and-effect – represents the next critical frontier in AI development.
What Constitutes “Reasoning” in AI?
Unlike human cognition, AI reasoning isn’t (yet) based on conscious understanding. Instead, it refers to a model’s ability to perform tasks that require logical steps, deduction, or manipulation of knowledge. Key indicators include:
- Multi-Step Problem Solving: Breaking down complex questions into intermediate steps (e.g., mathematical word problems, planning sequences).
- Inferential Capabilities: Drawing conclusions based on provided context, even if the answer isn’t explicitly stated.
- Causal Understanding: Recognizing cause-and-effect relationships within data or scenarios.
- Counterfactual Thinking: Evaluating “what if” scenarios based on changes to initial conditions.
- Generalization: Applying learned principles to new, unseen situations effectively.
While modern LLMs exhibit flashes of these abilities, often stimulated by techniques like Chain-of-Thought prompting, consistent and reliable reasoning remains a significant challenge.
Current Approaches and Limitations
Several techniques aim to elicit or enhance reasoning in LLMs:
Chain-of-Thought (CoT) Prompting
Encouraging the model to “think step-by-step” explicitly guides it through intermediate reasoning phases, often improving performance on complex tasks.
Self-Consistency
Generating multiple reasoning paths (e.g., via CoT) and selecting the most frequent answer improves robustness.
Tree of Thoughts (ToT)
Allowing the model to explore multiple reasoning paths simultaneously and evaluate their promise, mimicking a more deliberative human thought process.
Retrieval-Augmented Generation (RAG)
While primarily for knowledge grounding, RAG systems implicitly require reasoning to synthesize retrieved information with the user’s query.
Despite progress, limitations persist. Models can struggle with abstract concepts, symbolic manipulation, robust causal inference, and avoiding logical fallacies, especially when faced with problems outside their training distribution patterns. They often produce plausible-sounding but incorrect reasoning paths.
The Strategic Importance of AI Reasoning
Developing AI systems with stronger reasoning capabilities unlocks transformative potential across industries:
- Scientific Discovery: Assisting researchers in formulating hypotheses, designing experiments, and analyzing complex datasets.
- Advanced Diagnostics: Enhancing medical diagnosis by synthesizing patient data, medical literature, and logical inference.
- Autonomous Systems: Enabling more robust decision-making for self-driving vehicles, robotics, and complex logistics.
- Personalized Education: Creating AI tutors that can understand student reasoning and provide tailored explanations.
- Complex Financial Modeling: Improving risk assessment and forecasting through deeper causal analysis.
Businesses that can leverage AI capable of reliable reasoning will gain significant advantages in efficiency, innovation, and strategic foresight.
The Path Forward
Achieving human-like reasoning in AI remains a long-term goal. Near-term progress involves:
- Developing new model architectures incorporating explicit reasoning modules.
- Creating more challenging benchmarks to evaluate reasoning capabilities accurately.
- Combining symbolic AI techniques (rule-based systems) with neural networks.
- Improving techniques like CoT, ToT, and RAG through better prompting and fine-tuning.
Conclusion: Cultivating Intelligent Systems
Reasoning is the cornerstone of higher intelligence. As we continue to develop generative AI, focusing on enhancing its reasoning abilities is paramount. This involves not only architectural innovation but also sophisticated interaction strategies, like advanced prompt engineering and the design of systems like RAG that facilitate grounded reasoning. Understanding the nuances of AI reasoning is essential for businesses aiming to deploy AI solutions that go beyond simple automation to tackle complex, dynamic challenges.
Solveion helps organizations understand and leverage the latest AI capabilities, including developing systems that facilitate more robust reasoning. Contact us to explore the possibilities.